MuMA-ToM: A Multimodal Benchmark for Advancing Multi-Agent Theory of Mind Reasoning in AI
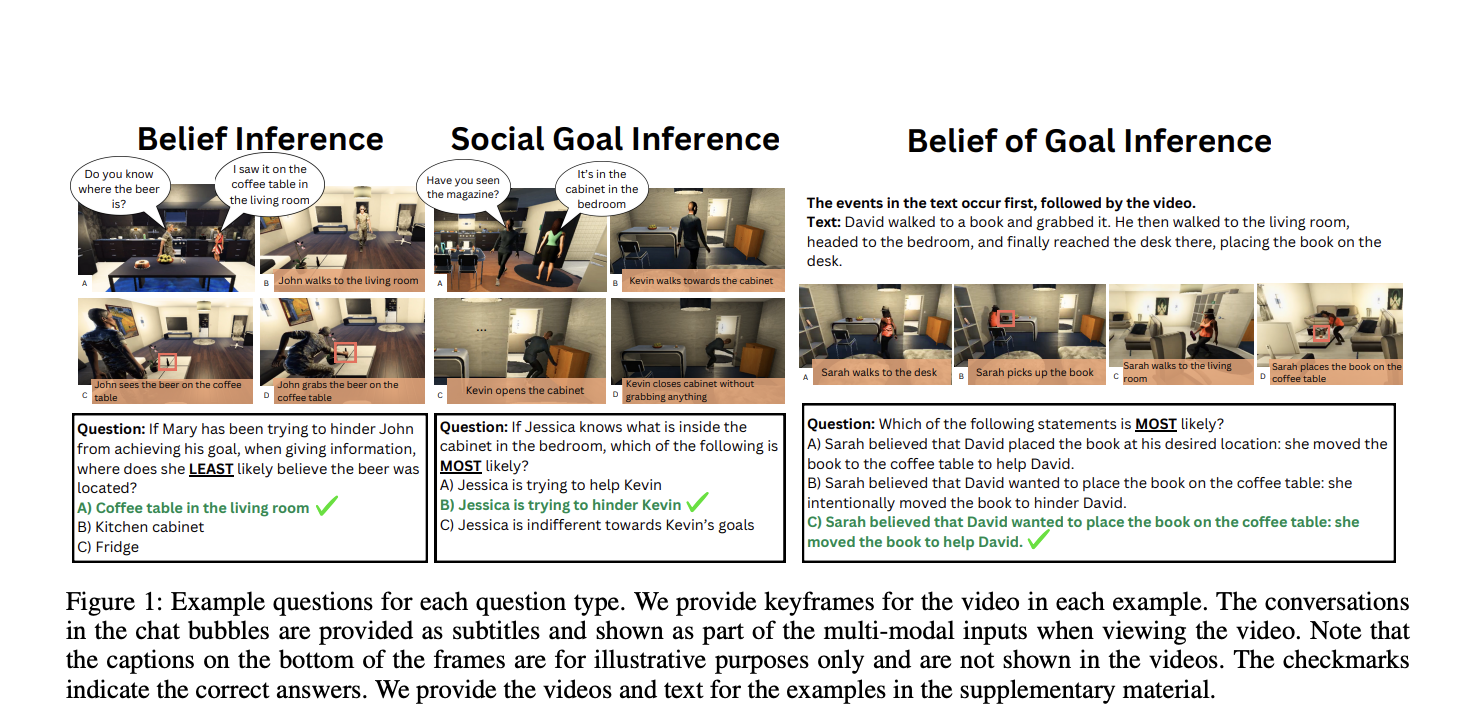
Understanding social interactions in complex real-world settings requires deep mental reasoning to infer the underlying mental states driving these interactions, known as the Theory of Mind (ToM). Social interactions are often multi-modal, involving actions, conversations, and past behaviors. For AI to effectively engage in human environments, it must grasp these mental states and their interrelations. Despite advances in machine ToM, current benchmarks mainly focus on individual mental states and lack multi-modal datasets for evaluating multi-agent ToM. This gap hinders the development of AI systems capable of understanding nuanced social interactions, which is crucial for safe human-AI interaction.
Researchers from Johns Hopkins University and the University of Virginia introduced MuMA-ToM, the first benchmark to assess multi-modal, multi-agent ToM reasoning in embodied interactions. MuMA-ToM presents videos and text describing real-life scenarios and poses questions about agents’ goals and beliefs about others’ goals. They validated MuMA-ToM through human experiments and introduced LIMP (Language model-based Inverse Multi-agent Planning), a novel ToM model. LIMP outperformed existing models, including GPT-4o and BIP-ALM, by integrating two-level reasoning and eliminating the need for symbolic representations. The work highlights the gap between human and machine ToM.
ToM benchmarks traditionally focus on single-agent reasoning, while multi-agent benchmarks often lack questions about inter-agent relationships. Existing ToM benchmarks usually rely on text or video, with few exceptions like MMToM-QA, which addresses single-agent activities in a multi-modal format. MuMA-ToM, however, introduces a benchmark for multi-agent ToM reasoning using text and video to depict realistic interactions. Unlike previous methods like BIP-ALM, which requires symbolic representations, the LIMP model enhances multi-agent planning and employs general, domain-invariant representations, improving ToM reasoning in multi-modal, multi-agent contexts.
The MuMA-ToM Benchmark evaluates models for understanding multi-agent social interactions using video and text. It features 225 interactions and 900 questions focused on three ToM concepts: belief inference, social goal inference, and belief of goal inference. The interactions are procedurally generated with distinct multimodal inputs, challenging models to effectively fuse this information. Based on the I-POMDP framework, the benchmark employs LIMP, which integrates vision-language and language models to infer mental states. Human accuracy is high, but even top models like Gemini 1.5 Pro and Llava 1.6 need to catch up.
In experiments, 18 participants from Prolific answered 90 randomly selected questions from the MuMA-ToM benchmark, achieving a high accuracy rate of 93.5%. State-of-the-art models, including Gemini 1.5 Pro and Llava 1.6, performed significantly worse, with the best model accuracy at 56.4%. The LIMP model outperformed others with a 76.6% accuracy by effectively integrating multimodal inputs and using natural language for action inference. However, LIMP’s limitations include susceptibility to visual hallucinations and lack of explicit multi-level reasoning. The benchmark is currently limited to two-agent interactions in synthetic household settings.
In conclusion, MuMA-ToM is the first multimodal Theory of Mind benchmark for evaluating mental reasoning in complex multi-agent interactions. MuMA-ToM uses video and text inputs to assess understanding of goals and beliefs in realistic household settings. The study systematically evaluated human performance and tested state-of-the-art models, proposing a model LIMP (Language model-based Inverse Multi-agent Planning). LIMP outperformed existing models, including GPT-4o and Gemini-1.5 Pro. Future work will extend the benchmark to more complex real-world scenarios, including interactions involving multiple agents and real-world videos.
Check out the Paper. All credit for this research goes to the researchers of this project. Also, don’t forget to follow us on Twitter and LinkedIn. Join our Telegram Channel. If you like our work, you will love our newsletter..
Don’t Forget to join our 50k+ ML SubReddit
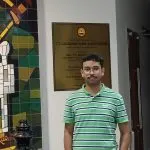
Sana Hassan, a consulting intern at Marktechpost and dual-degree student at IIT Madras, is passionate about applying technology and AI to address real-world challenges. With a keen interest in solving practical problems, he brings a fresh perspective to the intersection of AI and real-life solutions.