Demystifying Generative Artificial Intelligence: An In-Depth Dive into Diffusion Models and Visual Computing Evolution
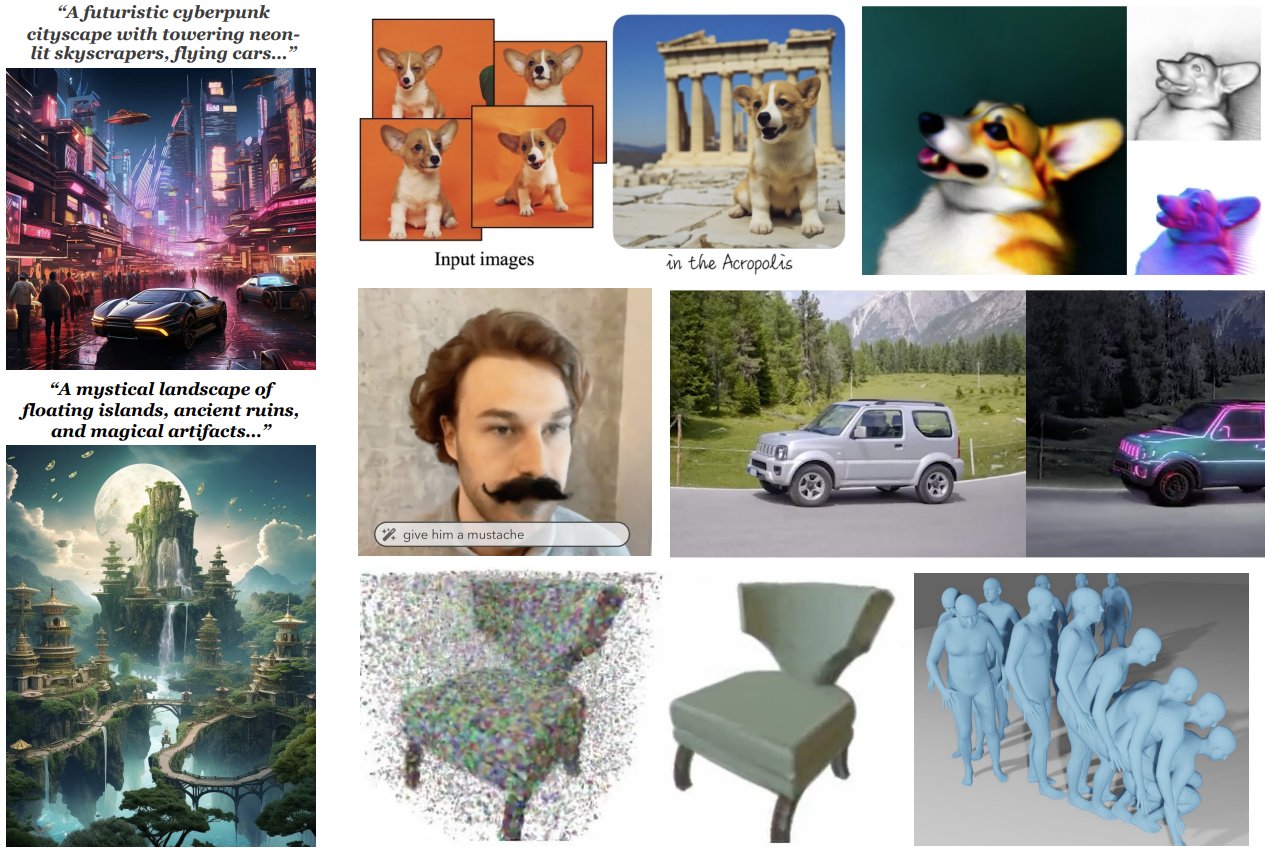
To combine computer-generated visuals or deduce the physical characteristics of a scene from pictures, computer graphics, and 3D computer vision groups have been working to create physically realistic models for decades. Several industries, including visual effects, gaming, image and video processing, computer-aided design, virtual and augmented reality, data visualization, robotics, autonomous vehicles, and remote sensing, among others, are built on this methodology, which includes rendering, simulation, geometry processing, and photogrammetry. An entirely new way of thinking about visual computing has emerged with the rise of generative artificial intelligence (AI). With only a written prompt or high-level human instruction as input, generative AI systems enable the creation and manipulation of photorealistic and styled photos, movies, or 3D objects.
These technologies automate several time-consuming tasks in visual computing that were previously only available to specialists with in-depth topic expertise. Foundation models for visual computing, such as Stable Diffusion, Imagen, Midjourney, or DALL-E 2 and DALL-E 3, have opened the unparalleled powers of generative AI. These models have “seen it all” after being trained on hundreds of millions to billions of text-image pairings, and they are incredibly vast, with just a few billion learnable parameters. These models were the basis for the generative AI tools mentioned above and were trained on an enormous cloud of powerful graphics processing units (GPUs).
The diffusion models based on convolutional neural networks (CNN) frequently used to generate images, videos, and 3D objects integrate text calculated using transformer-based architectures, such as CLIP, in a multi-modal fashion. There is still room for the academic community to make significant contributions to the development of these tools for graphics and vision, even though well-funded industry players have used a significant amount of resources to develop and train foundation models for 2D image generation. For example, it needs to be clarified how to adapt current picture foundation models for use in other, higher-dimensional domains, such as video and 3D scene creation.
A need for more specific kinds of training data mostly causes this. For instance, there are many more examples of low-quality and generic 2D photos on the web than of high-quality and varied 3D objects or settings. Furthermore, scaling 2D image creation systems to accommodate greater dimensions, as necessary for video, 3D scene, or 4D multi-view-consistent scene synthesis, is not immediately apparent. Another example of a current limitation is computation: even though an enormous amount of (unlabeled) video data is available on the web, current network architectures are frequently too inefficient to be trained in a reasonable amount of time or on a reasonable amount of compute resources. This results in diffusion models being rather slow at inference time. This is due to their networks’ large size and iterative nature.
Despite the unresolved issues, the number of diffusion models for visual computing has increased dramatically in the past year (see illustrative examples in Fig. 1). The objectives of this state-of-the-art report (STAR) developed by researchers from multiple universities are to offer an organized review of the numerous recent publications focused on applications of diffusion models in visual computing, to teach the principles of diffusion models, and to identify outstanding issues.
Check out the Paper. All Credit For This Research Goes To the Researchers on This Project. Also, don’t forget to join our 31k+ ML SubReddit, 40k+ Facebook Community, Discord Channel, and Email Newsletter, where we share the latest AI research news, cool AI projects, and more.
If you like our work, you will love our newsletter..
We are also on WhatsApp. Join our AI Channel on Whatsapp..
Aneesh Tickoo is a consulting intern at MarktechPost. He is currently pursuing his undergraduate degree in Data Science and Artificial Intelligence from the Indian Institute of Technology(IIT), Bhilai. He spends most of his time working on projects aimed at harnessing the power of machine learning. His research interest is image processing and is passionate about building solutions around it. He loves to connect with people and collaborate on interesting projects.