A New AI Research Releases SWIM-IR: A Large-Scale Synthetic Multilingual Retrieval Dataset with 28 Million Training Pairs over 33 Languages
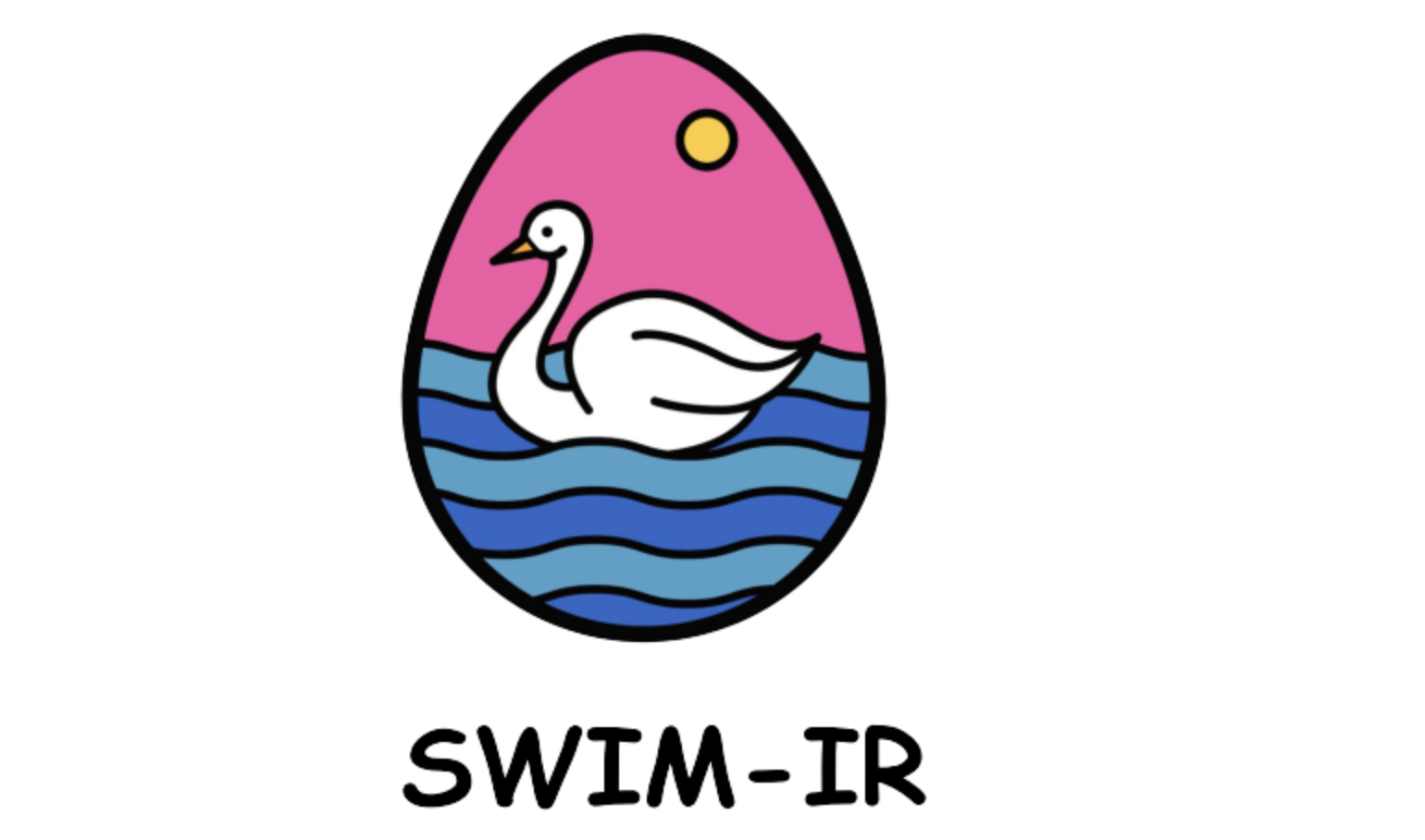
Researchers from Google Research, Google DeepMind, and the University of Waterloo introduce SWIM-IR, a synthetic retrieval training dataset encompassing 33 languages, addressing the challenge of limited human-labeled training pairs in multilingual retrieval. Leveraging the SAP (summarize-then-ask prompting) method, SWIM-IR is constructed to enable synthetic fine-tuning of multilingual dense retrieval models without human supervision. SWIM-X models, trained on SWIM-IR, demonstrate competitiveness with human-supervised thick retrieval models across various benchmarks, including XOR-Retrieve, XTREME-UP, and MIRACL.
The study addresses limitations in multilingual dense retrieval models. Existing multilingual retrieval models face challenges due to scarce or uneven training data. SWIM-IR employs SAP to assist LLMs in generating informative queries in the target language. SWIM-X models, trained on SWIM-IR, exhibit competitive performance with human-supervised models across various benchmarks, highlighting the potential of synthetic datasets as a cost-effective alternative to human-labeled training data for multilingual dense retrieval models.
The research addresses the limited success of multilingual dense retrieval models, attributing it to insufficient supervised training data for non-English languages. This synthetic dataset enables fine-tuning of multilingual dense retrieval models, evaluated on benchmarks like XOR-Retrieve, XTREME-UP, and MIRACL. Results demonstrate SWIM-IR’s efficacy in substituting expensive human-labeled training data, establishing competitive performance for multilingual dense retrieval models against human-supervised counterparts.
SWIM-IR, a synthetic retrieval training dataset spanning 33 languages, was generated through the SAP technique. Employing SWIM-IR, the study explores the synthetic fine-tuning of multilingual dense retrieval models, adapting the Dense Passage Retrieval (DPR) model. Utilizing the T5X Retrieval framework, it replicates mContriever and mDPR zero-shot baselines by initializing from a multilingual T5-base checkpoint and fine-tuning on the English MS MARCO dataset. Pretraining on the mC4 dataset and employing contrastive loss for in-batch negatives, the researchers use the PaLM 2 Small model for cross-language query generation.
Straight-turned on synthetic training data from SWIM-IR, SWIM-X models exhibit competitive performance in multilingual dense retrieval tasks. SWIM-X (7M) outperforms mContriever-X, the best-fine-tuned model, by 7.1 points on Recall5kt in the XOR-Retrieve benchmark. Even the limited-budget baseline, SWIM-X (500k), surpasses mContriever-X by 3.6 points. SWIM-X (180K) competes well on the MIRACL benchmark, outperforming the best zero-shot model by 6.6 points on nDCG10, although it falls short of mContriever-X, which benefits from human-labeled training pairs with hard negatives. Synthetic baselines, SWIM-X (120K) and SWIM-X (120K)MT show promising results in cross-lingual supervised baselines, outperforming existing models in terms of Recall5kt. The study emphasizes the importance of optimized training techniques, including better sampling hard negatives with SWIM-IR, to further enhance the performance of synthetic models.
The SWIM-IR dataset employed in the study exhibits limitations, including decontextualization, code-switching, passage quality and length, and factual inconsistencies in LLM generation. The study acknowledges that LLMs may generate text lacking sufficient grounding to knowledge sources, posing risks of misinformation and hallucination in generated outputs. While these limitations may impact the quality and accuracy of generated queries, they do not directly affect the downstream multilingual retrieval task. However, it does not extensively discuss the methods’ limitations, such as the SAP approach or the fine-tuning process.
SWIM-IR is a synthetic multilingual retrieval training dataset created using the SAP approach to generate informative queries in multiple languages. With 28 million query-passage training pairs across 33 languages, SWIM-IR facilitates fine-tuning multilingual dense retrieval models without requiring human-labeled training data. The resulting SWIM-X models exhibit competitive performance in multilingual retrieval tasks, outperforming existing recall and mean reciprocal rank models on both cross-lingual and monolingual benchmarks. It underscores SWIM-IR’s potential as a cost-effective substitute for expensive human-labeled retrieval training data, enabling the development of robust multilingual dense retrieval models.
Check out the Paper and Github. All credit for this research goes to the researchers of this project. Also, don’t forget to join our 33k+ ML SubReddit, 41k+ Facebook Community, Discord Channel, and Email Newsletter, where we share the latest AI research news, cool AI projects, and more.
If you like our work, you will love our newsletter..
Sana Hassan, a consulting intern at Marktechpost and dual-degree student at IIT Madras, is passionate about applying technology and AI to address real-world challenges. With a keen interest in solving practical problems, he brings a fresh perspective to the intersection of AI and real-life solutions.