Max Planck Researchers Introduce PoseGPT: An Artificial Intelligence Framework Employing Large Language Models (LLMs) to Understand and Reason about 3D Human Poses from Images or Textual Descriptions
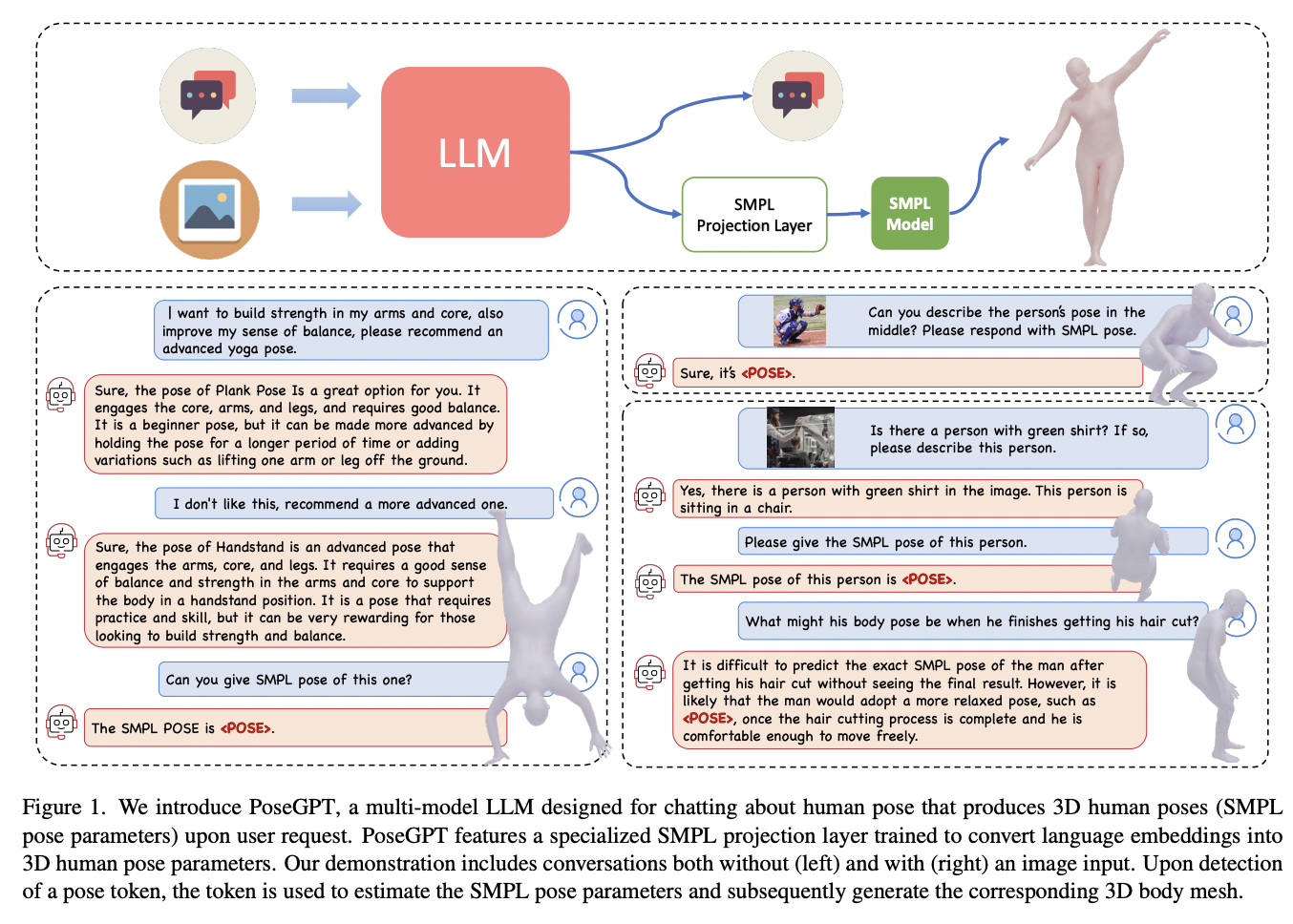
Human posture is crucial in overall health, well-being, and various aspects of life. It encompasses the alignment and positioning of the body while sitting, standing, or lying down. Good posture supports the optimal alignment of muscles, joints, and ligaments, reducing the risk of muscular imbalances, joint pain, and overuse injuries. It helps distribute the body’s weight evenly, preventing excessive stress on specific body parts.
Proper posture allows for better lung expansion and facilitates adequate breathing. Slouching or poor posture can compress the chest cavity, restricting lung capacity and hindering efficient breathing. Additionally, good posture supports healthy circulation throughout the body. Research suggests that maintaining good posture can positively influence mood and self-confidence. Adopting an upright and open posture is associated with increased assertiveness, positivity, and reduced stress levels.
A team of researchers from Max Plank Institute for Intelligent Systems, ETH Zurich, Meshcapade, and Tsinghua University built a framework employing a Large Language Model called PoseGPT to understand and reason about 3D human poses from images or textual descriptions. Traditional human pose estimation methods, like image-based or text-based, often need more holistic scene comprehension and nuanced reasoning, leading to a disconnect between visual data and its real-world implications. PoseGPT addresses these limitations by embedding SMPL poses as a distinct signal token within a multimodal LLM by enabling the direct generation of 3D body poses from both textual and visual inputs.
Their method embeds SMPL poses as a unique token by prompting the LLM to output these when queried about SMPL pose-related questions. They extracted the language embedding from this token and used an MLP (multi-layer perceptron) to predict the SMPL pose parameters directly. This enables the model to take either text or images as input and output 3D body poses.
They evaluated PoseGPT on various diverse tasks, like the traditional task of 3D human pose estimation from a single image and pose generation from text descriptions. The metric accuracy on these classical tasks still needs to match that of specialized methods, but they see this as a first proof of concept. More importantly, once the LLMs understand SMPL poses, they can use their inherent world knowledge to relate and reason about human poses without requiring extensive additional data or training.
Contrary to conventional approaches in pose regression, their methodology does not involve providing the multimodal LLM with a cropped bounding box surrounding the individual. Instead, the model is exposed to the entire scene, enabling them to formulate queries regarding the individuals and their respective poses within that context.
Once the LLM grasps the concept of 3D body pose, it gains the dual ability to generate human poses and to comprehend the world. This enables it to reason through complex verbal and visual inputs and develop human poses. This leads to the introduction of novel tasks made possible by this capability and benchmarks to assess performance to any model.
Check out the Paper and Project. All credit for this research goes to the researchers of this project. Also, don’t forget to join our 33k+ ML SubReddit, 41k+ Facebook Community, Discord Channel, and Email Newsletter, where we share the latest AI research news, cool AI projects, and more.
If you like our work, you will love our newsletter..
Arshad is an intern at MarktechPost. He is currently pursuing his Int. MSc Physics from the Indian Institute of Technology Kharagpur. Understanding things to the fundamental level leads to new discoveries which lead to advancement in technology. He is passionate about understanding the nature fundamentally with the help of tools like mathematical models, ML models and AI.