Google DeepMind unveils ‘superhuman’ AI system that excels in fact-checking, saving costs and improving accuracy
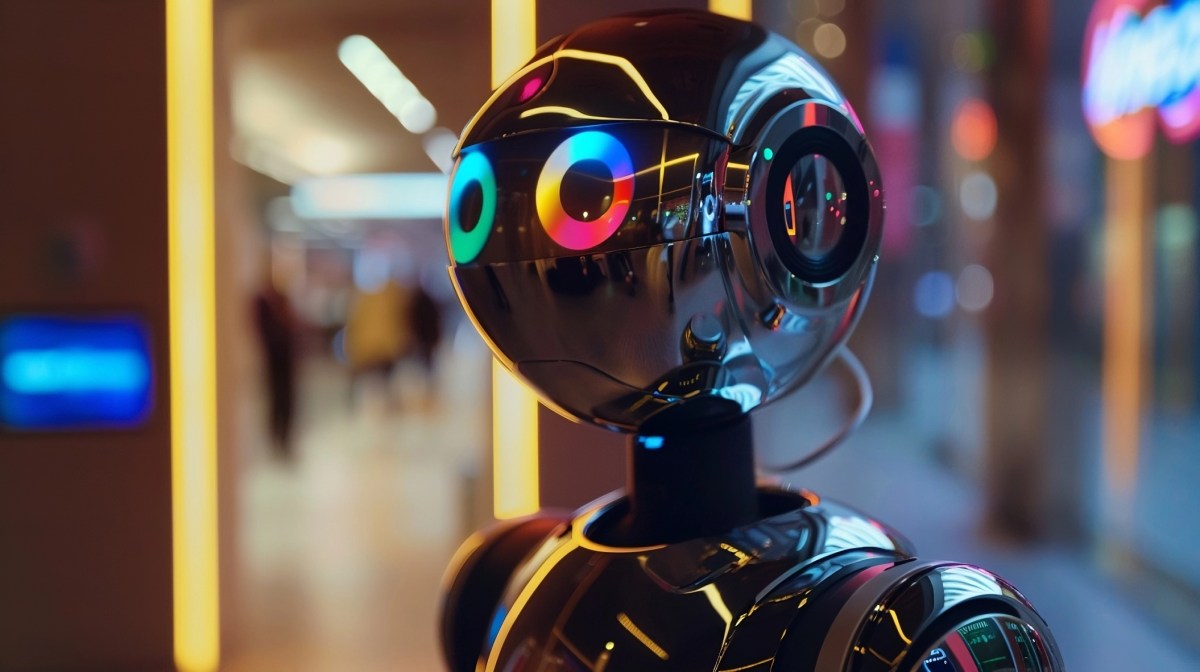
[ad_1]
Join us in Atlanta on April 10th and explore the landscape of security workforce. We will explore the vision, benefits, and use cases of AI for security teams. Request an invite here.
A new study from Google’s DeepMind research unit has found that an artificial intelligence system can outperform human fact-checkers when evaluating the accuracy of information generated by large language models.
The paper, titled “Long-form factuality in large language models” and published on the pre-print server arXiv, introduces a method called Search-Augmented Factuality Evaluator (SAFE). SAFE uses a large language model to break down generated text into individual facts, and then uses Google Search results to determine the accuracy of each claim.
“SAFE utilizes an LLM to break down a long-form response into a set of individual facts and to evaluate the accuracy of each fact using a multi-step reasoning process comprising sending search queries to Google Search and determining whether a fact is supported by the search results,” the authors explained.
‘Superhuman’ performance sparks debate
The researchers pitted SAFE against human annotators on a dataset of roughly 16,000 facts, finding that SAFE’s assessments matched the human ratings 72% of the time. Even more notably, in a sample of 100 disagreements between SAFE and the human raters, SAFE’s judgment was found to be correct in 76% of cases.
VB Event
The AI Impact Tour – Atlanta
Request an invite
While the paper asserts that “LLM agents can achieve superhuman rating performance,” some experts are questioning what “superhuman” really means here.
Gary Marcus, a well-known AI researcher and frequent critic of overhyped claims, suggested on Twitter that in this case, “superhuman” may simply mean “better than an underpaid crowd worker, rather a true human fact checker.”
“That makes the characterization misleading,” he said. “Like saying that 1985 chess software was superhuman.”
Marcus raises a valid point. To truly demonstrate superhuman performance, SAFE would need to be benchmarked against expert human fact-checkers, not just crowdsourced workers. The specific details of the human raters, such as their qualifications, compensation, and fact-checking process, are crucial for properly contextualizing the results.
Cost savings and benchmarking top models
One clear advantage of SAFE is cost — the researchers found that using the AI system was about 20 times cheaper than human fact-checkers. As the volume of information generated by language models continues to explode, having an economical and scalable way to verify claims will be increasingly vital.
The DeepMind team used SAFE to evaluate the factual accuracy of 13 top language models across 4 families (Gemini, GPT, Claude, and PaLM-2) on a new benchmark called LongFact. Their results indicate that larger models generally produced fewer factual errors.
However, even the best-performing models generated a significant number of false claims. This underscores the risks of over-relying on language models that can fluently express inaccurate information. Automatic fact-checking tools like SAFE could play a key role in mitigating those risks.
Transparency and human baselines are crucial
While the SAFE code and LongFact dataset have been open-sourced on GitHub, allowing other researchers to scrutinize and build upon the work, more transparency is still needed around the human baselines used in the study. Understanding the specifics of the crowdworkers’ background and process is essential for assessing SAFE’s capabilities in proper context.
As the tech giants race to develop ever more powerful language models for applications ranging from search to virtual assistants, the ability to automatically fact-check the outputs of these systems could prove pivotal. Tools like SAFE represent an important step towards building a new layer of trust and accountability.
However, it’s crucial that the development of such consequential technologies happens in the open, with input from a broad range of stakeholders beyond the walls of any one company. Rigorous, transparent benchmarking against human experts — not just crowdworkers — will be essential to measure true progress. Only then can we gauge the real-world impact of automated fact-checking on the fight against misinformation.
[ad_2]
Source link