Unlocking the potential of AI on edge devices
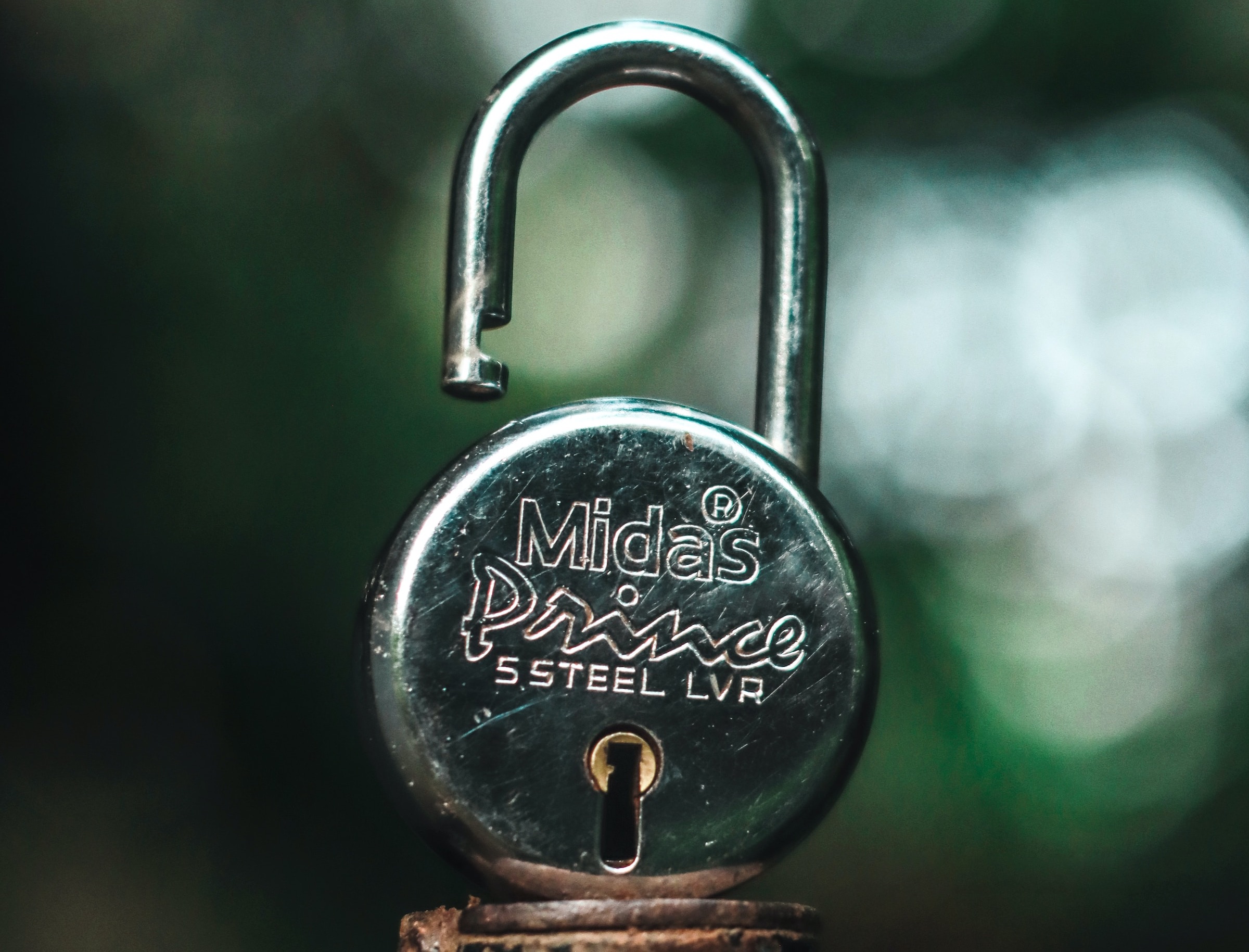
In an interview at AI & Big Data Expo, Alessandro Grande, Head of Product at Edge Impulse, discussed issues around developing machine learning models for resource-constrained edge devices and how to overcome them.
During the discussion, Grande provided insightful perspectives on the current challenges, how Edge Impulse is helping address these struggles, and the tremendous promise of on-device AI.
Key hurdles with edge AI adoption
Grande highlighted three primary pain points companies face when attempting to productise edge machine learning models, including difficulties determining optimal data collection strategies, scarce AI expertise, and cross-disciplinary communication barriers between hardware, firmware, and data science teams.
“A lot of the companies building edge devices are not very familiar with machine learning,” says Grande. “Bringing those two worlds together is the third challenge, really, around having teams communicate with each other and being able to share knowledge and work towards the same goals.”
Strategies for lean and efficient models
When asked how to optimise for edge environments, Grande emphasised first minimising required sensor data.
“We are seeing a lot of companies struggle with the dataset. What data is enough, what data should they collect, what data from which sensors should they collect the data from. And that’s a big struggle,” explains Grande.
Selecting efficient neural network architectures helps, as does compression techniques like quantisation to reduce precision without substantially impacting accuracy. Always balance sensor and hardware constraints against functionality, connectivity needs, and software requirements.
Edge Impulse aims to enable engineers to validate and verify models themselves pre-deployment using common ML evaluation metrics, ensuring reliability while accelerating time-to-value. The end-to-end development platform seamlessly integrates with all major cloud and ML platforms.
Transformative potential of on-device intelligence
Grande highlighted innovative products already leveraging edge intelligence to provide personalised health insights without reliance on the cloud, such as sleep tracking with Oura Ring.
“It’s sold over a billion pieces, and it’s something that everybody can experience and everybody can get a sense of really the power of edge AI,” explains Grande.
Other exciting opportunities exist around preventative industrial maintenance via anomaly detection on production lines.
Ultimately, Grande sees massive potential for on-device AI to greatly enhance utility and usability in daily life. Rather than just raw data, edge devices can interpret sensor inputs to provide actionable suggestions and responsive experiences not previously possible—heralding more useful technology and improved quality of life.
Unlocking the potential of AI on edge devices hinges on overcoming current obstacles inhibiting adoption. Grande and other leading experts provided deep insights at this year’s AI & Big Data Expo on how to break down the barriers and unleash the full possibilities of edge AI.
“I’d love to see a world where the devices that we were dealing with were actually more useful to us,” concludes Grande.
Watch our full interview with Alessandro Grande below:
(Photo by Niranjan _ Photographs on Unsplash)
See also: AI & Big Data Expo: Demystifying AI and seeing past the hype
Want to learn more about AI and big data from industry leaders? Check out AI & Big Data Expo taking place in Amsterdam, California, and London. The comprehensive event is co-located with Cyber Security & Cloud Expo and Digital Transformation Week.
Explore other upcoming enterprise technology events and webinars powered by TechForge here.